MIT created an AI/ML system last year that can learn and adapt to new information while on the job, not only during its initial training period. These “liquid” neural networks (in the Bruce Lee sense) essentially play 4D chess with time-series data, making them perfect for application in time-sensitive tasks such as pacemaker monitoring, weather forecasting, investment forecasting, or autonomous vehicle navigation. However, data flow has become a barrier, and expanding these systems has become prohibitively costly in terms of computing.
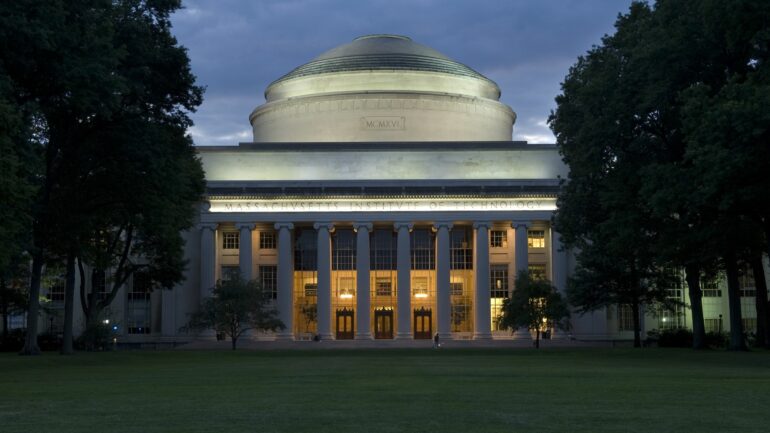